Package: miWQS 0.5.0
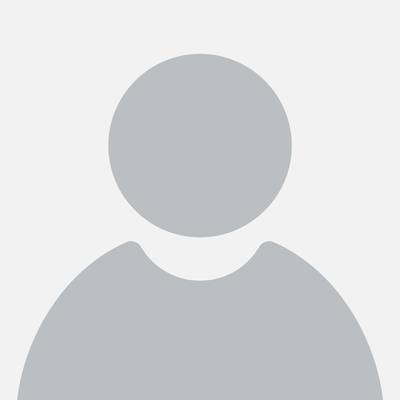
Paul M. Hargarten
miWQS: Multiple Imputation Using Weighted Quantile Sum Regression
The miWQS package handles the uncertainty due to below the detection limit in a correlated component mixture problem. Researchers want to determine if a set/mixture of continuous and correlated components/chemicals is associated with an outcome and if so, which components are important in that mixture. These components share a common outcome but are interval-censored between zero and low thresholds, or detection limits, that may be different across the components. This package applies the multiple imputation (MI) procedure to the weighted quantile sum regression (WQS) methodology for continuous, binary, or count outcomes (Hargarten & Wheeler (2020) <doi:10.1016/j.envres.2020.109466>). The imputation models are: bootstrapping imputation (Lubin et al (2004) <doi:10.1289/ehp.7199>), univariate Bayesian imputation (Hargarten & Wheeler (2020) <doi:10.1016/j.envres.2020.109466>), and multivariate Bayesian regression imputation.
Authors:
miWQS_0.5.0.tar.gz
miWQS_0.5.0.zip(r-4.5)miWQS_0.5.0.zip(r-4.4)miWQS_0.5.0.zip(r-4.3)
miWQS_0.5.0.tgz(r-4.5-any)miWQS_0.5.0.tgz(r-4.4-any)miWQS_0.5.0.tgz(r-4.3-any)
miWQS_0.5.0.tar.gz(r-4.5-noble)miWQS_0.5.0.tar.gz(r-4.4-noble)
miWQS_0.5.0.tgz(r-4.4-emscripten)miWQS_0.5.0.tgz(r-4.3-emscripten)
miWQS.pdf |miWQS.html✨
miWQS/json (API)
NEWS
# Install 'miWQS' in R: |
install.packages('miWQS', repos = c('https://phargarten2.r-universe.dev', 'https://cloud.r-project.org')) |
Bug tracker:https://github.com/phargarten2/miwqs/issues
- simdata87 - Simulated Dataset 87
- wqs.pool.test - Combining WQS Regression Estimates
Last updated 1 years agofrom:501aba6728. Checks:1 OK, 7 NOTE. Indexed: yes.
Target | Result | Latest binary |
---|---|---|
Doc / Vignettes | OK | Feb 01 2025 |
R-4.5-win | NOTE | Feb 01 2025 |
R-4.5-mac | NOTE | Feb 01 2025 |
R-4.5-linux | NOTE | Feb 01 2025 |
R-4.4-win | NOTE | Feb 01 2025 |
R-4.4-mac | NOTE | Feb 01 2025 |
R-4.3-win | NOTE | Feb 01 2025 |
R-4.3-mac | NOTE | Feb 01 2025 |
Exports:analyze.individuallycombine.AICdo.many.wqsestimate.wqsestimate.wqs.formulaimpute.bootimpute.Lubinimpute.multivariate.bayesianimpute.subimpute.univariate.bayesian.mimake.quantile.matrixpool.mi
Dependencies:backportsbase64encbslibcachemcheckmatecliclustercodacolorspacecondMVNormcpp11data.tabledigestdplyrevaluatefansifarverfastmapfontawesomeforeignFormulafsgenericsggplot2glm2gluegmmgridExtragtablehighrHmischtmlTablehtmltoolshtmlwidgetsinvgammaisobandjquerylibjsonliteknitrlabelinglatticelifecyclemagrittrMASSMatrixMatrixModelsmatrixNormalmcmcMCMCpackmemoisemgcvmimemunsellmvtnormnlmennetpillarpkgconfigpurrrquantregR6rappdirsRColorBrewerrlangrlistrmarkdownrpartRsolnprstudioapisandwichsassscalesSparseMstringistringrsurvivaltibbletidyrtidyselecttinytextmvmixnormtmvtnormtruncnormutf8vctrsviridisviridisLitewithrxfunXMLyamlzoo
Readme and manuals
Help Manual
Help page | Topics |
---|---|
Performing Individual Chemical Analysis | analyze.individually |
check_constants: K, B, T, n.burn all used this function | check_constants |
Finding WQS Coefficients | coef.wqs |
Combining AICs | combine.AIC |
Performing Many WQS Regressions | do.many.wqs |
Weighted Quantile Sum (WQS) Regression | estimate.wqs |
Formula for WQS Regression | estimate.wqs.formula |
Bootstrapping Imputation for Many Chemicals | impute.boot |
Lubin et al. 2004: Bootstrapping Imputation for One Chemical | impute.Lubin |
Multivariate Bayesian Imputation | impute.multivariate.bayesian |
Imputing by Substitution | impute.sub |
Univariate Bayesian Imputation | impute.univariate.bayesian.mi |
Making Quantiles of Correlated Index | make.quantile.matrix |
Histograms of the Weights, Beta1, and WQS using 'ggplot' | plot.wqs |
Pooling Multiple Imputation Results | pool.mi |
Prints the fitted WQS model along with the mean weights. | print.wqs |
Simulated Dataset 87 | simdata87 |
Combining WQS Regression Estimates | wqs.pool.test |